浅谈对pytroch中torch.autograd.backward的思考
更新时间:2019年12月27日 08:26:39 作者:https://oldpan.me/archives/pytroch-torch-autograd-backward
这篇文章主要介绍了对pytroch中torch.autograd.backward的思考,文中通过示例代码介绍的非常详细,对大家的学习或者工作具有一定的参考学习价值,需要的朋友们下面随着小编来一起学习学习吧
反向传递法则是深度学习中最为重要的一部分,torch中的backward可以对计算图中的梯度进行计算和累积
这里通过一段程序来演示基本的backward操作以及需要注意的地方
1 2 3 4 5 6 7 8 9 10 11 12 13 14 15 16 17 18 19 20 21 22 23 24 25 26 27 28 29 30 31 32 33 34 35 36 37 38 39 40 41 42 43 44 45 46 47 48 49 50 51 52 53 54 55 56 57 58 59 60 61 62 63 64 65 66 67 68 69 70 71 72 73 74 75 76 77 78 79 80 81 82 83 84 85 86 87 88 89 90 91 92 93 94 95 96 | >>> import torch >>> from torch.autograd import Variable >>> x = Variable(torch.ones( 2 , 2 ), requires_grad = True ) >>> y = x + 2 >>> y.grad_fn Out[ 6 ]: <torch.autograd.function.AddConstantBackward at 0x229e7068138 > >>> y.grad >>> z = y * y * 3 >>> z.grad_fn Out[ 9 ]: <torch.autograd.function.MulConstantBackward at 0x229e86cc5e8 > >>> z Out[ 10 ]: Variable containing: 27 27 27 27 [torch.FloatTensor of size 2x2 ] >>> out = z.mean() >>> out.grad_fn Out[ 12 ]: <torch.autograd.function.MeanBackward at 0x229e86cc408 > >>> out.backward() # 这里因为out为scalar标量,所以参数不需要填写 >>> x.grad Out[ 19 ]: Variable containing: 4.5000 4.5000 4.5000 4.5000 [torch.FloatTensor of size 2x2 ] >>> out # out为标量 Out[ 20 ]: Variable containing: 27 [torch.FloatTensor of size 1 ] >>> x = Variable(torch.Tensor([ 2 , 2 , 2 ]), requires_grad = True ) >>> y = x * 2 >>> y Out[ 52 ]: Variable containing: 4 4 4 [torch.FloatTensor of size 3 ] >>> y.backward() # 因为y输出为非标量,求向量间元素的梯度需要对所求的元素进行标注,用相同长度的序列进行标注 Traceback (most recent call last): File "C:\Users\dell\Anaconda3\envs\my-pytorch\lib\site-packages\IPython\core\interactiveshell.py" , line 2862 , in run_code exec (code_obj, self .user_global_ns, self .user_ns) File "<ipython-input-53-95acac9c3254>" , line 1 , in <module> y.backward() File "C:\Users\dell\Anaconda3\envs\my-pytorch\lib\site-packages\torch\autograd\variable.py" , line 156 , in backward torch.autograd.backward( self , gradient, retain_graph, create_graph, retain_variables) File "C:\Users\dell\Anaconda3\envs\my-pytorch\lib\site-packages\torch\autograd\__init__.py" , line 86 , in backward grad_variables, create_graph = _make_grads(variables, grad_variables, create_graph) File "C:\Users\dell\Anaconda3\envs\my-pytorch\lib\site-packages\torch\autograd\__init__.py" , line 34 , in _make_grads raise RuntimeError( "grad can be implicitly created only for scalar outputs" ) RuntimeError: grad can be implicitly created only for scalar outputs >>> y.backward(torch.FloatTensor([ 0.1 , 1 , 10 ])) >>> x.grad #注意这里的0.1,1.10为梯度求值比例 Out[ 55 ]: Variable containing: 0.2000 2.0000 20.0000 [torch.FloatTensor of size 3 ] >>> y.backward(torch.FloatTensor([ 0.1 , 1 , 10 ])) >>> x.grad # 梯度累积 Out[ 57 ]: Variable containing: 0.4000 4.0000 40.0000 [torch.FloatTensor of size 3 ] >>> x.grad.data.zero_() # 梯度累积进行清零 Out[ 60 ]: 0 0 0 [torch.FloatTensor of size 3 ] >>> x.grad # 累积为空 Out[ 61 ]: Variable containing: 0 0 0 [torch.FloatTensor of size 3 ] >>> y.backward(torch.FloatTensor([ 0.1 , 1 , 10 ])) >>> x.grad Out[ 63 ]: Variable containing: 0.2000 2.0000 20.0000 [torch.FloatTensor of size 3 ] |
以上就是本文的全部内容,希望对大家的学习有所帮助,也希望大家多多支持脚本之家。
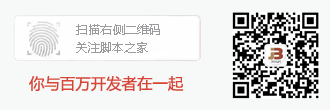
微信公众号搜索 “ 脚本之家 ” ,选择关注
程序猿的那些事、送书等活动等着你
本文来自互联网用户投稿,该文观点仅代表作者本人,不代表本站立场。本站仅提供信息存储空间服务,不拥有所有权,不承担相关法律责任。
如若内容造成侵权/违法违规/事实不符,请将相关资料发送至 reterry123@163.com 进行投诉反馈,一经查实,立即处理!
相关文章
python可视化分析的实现(matplotlib、seaborn、ggplot2)
这篇文章主要介绍了python可视化分析的实现(matplotlib、seaborn、ggplot2),文中通过示例代码介绍的非常详细,对大家的学习或者工作具有一定的参考学习价值,需要的朋友们下面随着小编来一起学习学习吧2021-02-02Python中利用Scipy包的SIFT方法进行图片识别的实例教程
SIFT算法可以检测图片中的局部特征,算法原理相当复杂...但是!Python强大的第三方包Scipy中带有实现SIFT算法的SIFT方法,我们只要拿来用就可以了,下面就为大家带来Python中利用Scipy包的SIFT方法进行图片识别的实例教程.2016-06-06Python Json模块中dumps、loads、dump、load函数介绍
本篇文章主要介绍了Python Json模块中dumps、loads、dump、load函数介绍,详细的介绍了这几种函数的用法,具有一定的参考价值,感兴趣的小伙伴们可以参考一下2018-05-05
最新评论